What does the Future of Data Science Look Like?

Image courtesy of Pexels.
In the early 2010s, data science became a buzzword that every company was using and as the need to process and understand large amounts of data continued to grow, so has the interest in the data science industry.
The first data scientists to enter the market had advanced backgrounds in statistics and analytics and were able to transfer these skills into data modeling.
These early data science pioneers paved the way for today and tomorrow’s data scientists. While the industry has seen a shift due to technological advancements, the need for data scientists is just as important as it ever was.
In this article, we’ll take a closer look at how the role of a data scientist has changed over the years and where we see it going into the future.
Here’s a quick look at the main topic areas we’ll cover:
- What does a data scientist do?
- How did data science start?
- Data scientists of the future
- How companies can use data science in the future
What does a data scientist do?
Data scientists are analysts that are responsible for collecting, analyzing, and interpreting large amounts of data. Individuals in the data science industry often have skill sets that span multiple technical aspects such as math, science, statistics, and technology.
Together, these skills allow data scientists to filter through data and pull out valuable insights that can help the business become more competitive in their markets.
Some of the common responsibilities of a data scientist include:
- Gathering and analyzing data
- Detect patterns within data sets
- Identify common trends
- Recognize important relationships
It’s important to note that the role of a data scientist may vary greatly from one company to the next. Some data scientists are tasked with designing new statistical models, while others are more focused on writing structured query language (SQL).
In many cases, the roles between a data scientist, analyst, and business intelligence can become blurred. Each of these roles plays a vital role in helping companies understand, report, and act on their data.
As technology makes it easier to create models, understand data trends, and deliver valuable data insights, the role of the data scientist will change. They may no longer have to specialize in mathematics or statistics, opening the door for analysts of all kinds to enter into the data science realm.
How Did Data Science Start?
Knowing that the role of data scientist wasn’t a known profession until the early 2010s, there weren’t many colleges or universities that offered degrees in data science. Data scientists began their careers as statistical or data analysts in many cases.
Individuals who were already specializing in these areas were the perfect people to shift into the world of data science because they already had the necessary technical knowledge. They could easily transfer their skills and begin to analyze data sets.
Early on, those who entered the data science industry would manually build and tune models—relying on their skills and knowledge to process data and identify important trends within the data sets.
To start, these early data scientists only had access to historical data. While this provided businesses with valuable insights about their business, customers, and market, it could never keep up with the ever-changing data in real-time.

Once the idea of big data became a regular practice, the manual data mining of the past became harder to manage. Companies started to require faster processing and more real-time insights to drive business decisions, which began to shift the role of the data scientist towards what it is today.
Data Scientists of The Future
The data science industry has begun to see a shift in the last few years. This shift is due to the many advancements in technology and the mass amounts of data being collected daily.

No longer are data scientists required to hand-build or hand-tuned models on their own. The emergence of big data has made accurately analyzing these data sets nearly impossible for individuals without the help of technology.
Luckily, technology has made these things more manageable in recent years while also producing more accurate insights – allowing data scientists to do more with their time.
The future data scientists will need to become familiar with new technology and have a deeper understanding between inputs and outputs – and then be able to communicate and present those in human-interpretable ways.
You can expect that these data scientists of the future will spend a large deal of their time designing experiments, confirming hypotheses, and writing SQLs to build features designed to improve model accuracy.
While there are plenty of data scientists who will still rely on their old models and skills to provide their companies with the information needed, the data scientists of the future will rely heavily on new technology to drive valuable insights and predictions.
As new data scientists enter the industry and companies continue to look for new ways to innovate and make the most out of their investments, the interest in data science technology will only continue to grow.
Another area where you can expect to see a shift in the data science world is education. Many college and university programs are already falling behind and will likely begin to improve their data science programs in the coming years.
But it’s almost important to consider that with the help of technological advancements, data scientists may no longer have to be individuals who have an advanced specialty in mathematics or statistics. Today, many of the available programs make it easier for anyone to collect, analyze, and report on business analytics.
Next, let’s take a closer look at how some of the recent technological advancements that are driving change in the data science industry.
Technology Advancements in the Data Science Industry
One of the most significant driving factors for change in the data science industry is related to new technology advancements. While some of the technology has existed for years, it’s finally becoming more widespread and reliable enough for companies to warrant the investment.
These technologies include things like artificial intelligence, automated machine learning, and deep learning capabilities. These tools provide data scientists with a way to improve their day-to-day processes and drive more efficiencies within their work.
This leads to more reliable, accurate data that the business can use to drive better customer experiences and stay competitive within the industry.
Let’s take a quick look at how each of these technologies is having an impact on the data science industry.
Artificial Intelligence
The recent advancements in artificial intelligence (AI) have had a big impact on many different roles and industries – and the data science field is no different.

AI makes it much easier for data scientists to collect and analyze large amounts of data quicker than ever before. This makes managing, understanding, and developing actionable insights from big data simple.
What once took one data scientist hours to analyze can now be done in mere minutes.
AI has provided businesses with the opportunity to understand customer behaviors and market trends at a larger scale than ever imaginable. For the data scientists of today and tomorrow, AI will play a huge part in their everyday activities and analysis.
Automated Machine Learning
Automated machine learning, or AutoML, is an aid to help companies develop better models for machine learning. It’s an advanced technology that takes the benefits of machine learning and automates them to do even more for businesses.
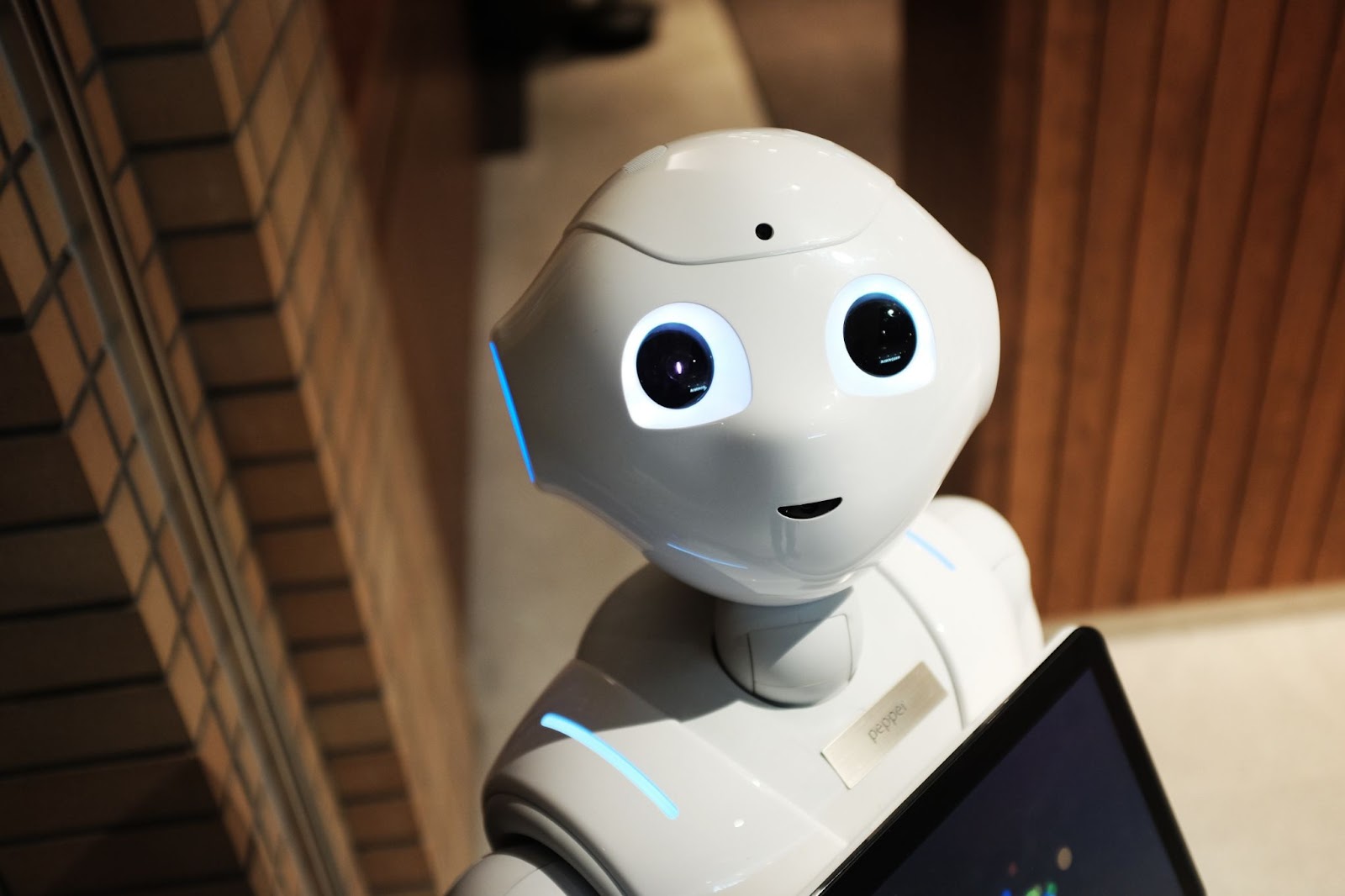
It will remove many of the tedious, time-consuming tasks that data scientists do on a regular basis and produce more accurate reports.
That being said, AutoML has the ability to change the way we see the data scientists of the future. While it will never fully replace the role of a data scientist, it will make it easier for people who may not have advanced knowledge in modeling or statistical analysis to enter the industry.
AutoML will make it easier for companies of all shapes and sizes to analyze and understand large data sets at scale. Individuals with analyst skill sets that may not have formal data science training can now use technology like AutoML to drive more actionable data insights.
Deep Learning
Deep learning is a subset of machine learning that trains computers to perform human-like tasks. Some of the things that computers can be trained to learn are things like speech recognition, image identification, and prediction making.

This type of technology learns on its own and begins to identify key patterns that present themselves within the data. It automates the machine learning process by actually adjusting and learning as it filters through data.
With deep learning technology, you can stay ahead of changing data sets and react in real-time. Having this insight can allow you to integrate more personalization into customer interactions or better predict their future needs.
How Companies Can Use Data Science In the Future
There are many different ways that companies can use data science to help improve their daily activities. From better understanding their customers to image and speech recognition – data scientists can help make decision-making easier with the backing of data.
When it comes to how companies can use data science, the possibilities are endless. It can help identify areas of revenue opportunity, help to understand customer needs better, or help eliminate waste within your day-to-day work.
These are a few specific examples of how companies can use data science:
- Fraud and risk detection
- Imaging analysis and diagnosis in the healthcare industry
- Image recognition and speech recognition
- Individualize the gaming industry
- Assess customer lifetime value
- Improved eCommerce experience
- Machine learning in manufacturing plants
Companies that take advantage of the data they're already collecting and make the investment in an educated data science team will set themselves up for success. It will give them a competitive advantage that will help them to improve and identify new opportunities for growth continuously.
The Future of Data Science is Bright
Mass amounts of data are being collected every day, and that’s not going to change anytime soon. Having the ability to understand this data is essential to the growth of your business. It will allow you to continue learning and improving all areas of your business and help you make data-driven decisions that make an impact.
Data science isn’t going anywhere. If you haven’t already made the decision to do more with your data, now’s the time to get started.